Data Mesh vs. Data Fabric: Understanding the Differences
- Ankit Mittal
- Jan 24, 2024
- 3 min read
In the ever-evolving landscape of data management and analytics, two distinct paradigms have emerged to address the diverse needs and complexities of handling vast amounts of information: Data Fabric, and Data Mesh. These approaches represent unique methodologies for storing, processing, and extracting insights from data, each catering to different use cases and requirements. Data Fabrics consolidate data from legacy systems, data warehouses, SQL databases, and data lakes, thereby offering a comprehensive perspective on business performance. In contrast, Data Mesh introduces a paradigm shift, advocating for decentralized data ownership and emphasizing data as a product. This introductory exploration sets the stage for understanding how these three methodologies shape the data landscape, empowering organizations to harness the full potential of their data assets.
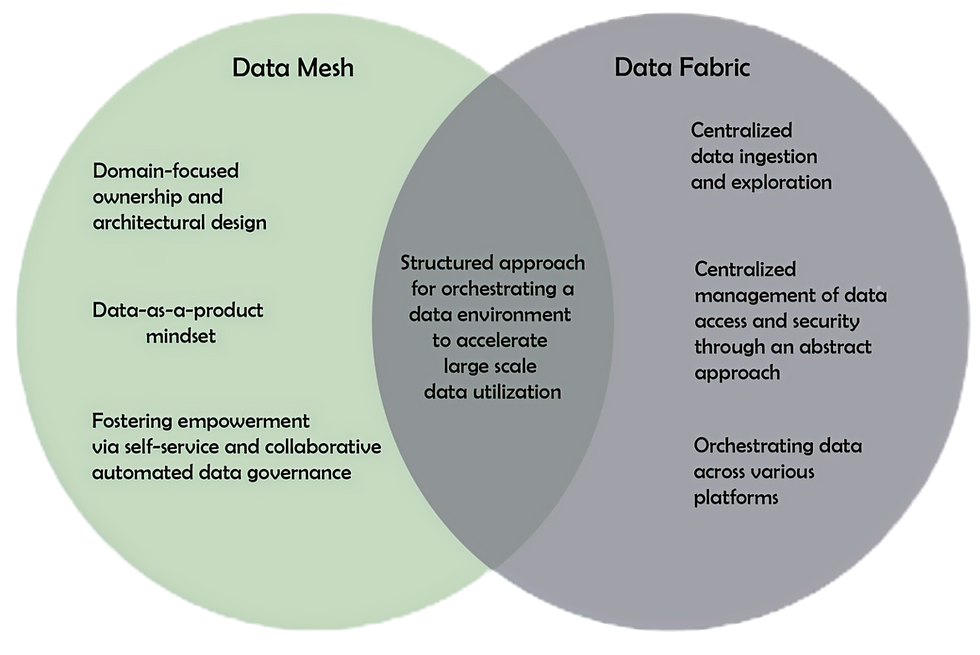
Data Mesh
A data mesh is an architectural framework that addresses complex data security issues by implementing distributed, decentralized ownership. In organizations, data is sourced from various business segments and needs to be integrated for analytical purposes. The data mesh architecture operates under four principles, efficiently connecting these diverse data sources by enforcing centralized data sharing and governance rules. This approach empowers business units to exert control over data access, defining who can access it and in what formats. While a data mesh introduces architectural complexities, it enhances data accessibility, security, and scalability, offering an effective solution to these challenges. JP Morgan Chase & Co. CIO, James Reid wanted to refresh the JPMC platform with three main goals: cut costs, accelerate time to value, and data re-used as a fundamental value ingredient. To accomplish these tasks, they implemented a data mesh. JPMC implemented a federated lake (mesh) formation approach, allowing each line of business (LoB) to create multiple data producer and consumer accounts, which roll up to a central master line of business lake formation accounts. These data products are interconnected in a federated model, consolidating into a master Glue Catalog for authorized users to locate specific data elements. While the system delegates much management responsibility to individual lines of business, a centralized master catalog ensures federated and automated governance, overseen by the Chief Data Officer at JPMC for governance and compliance across the federation.
Data Fabric
By making use of data services and APIs, data fabrics orchestrate the consolidation of data from legacy systems, data lakes, data warehouses, SQL databases, and applications, thereby offering a comprehensive perspective on business performance. Unlike individual data storage systems, their objective is to enhance the fluidity of data across various environments, mitigating the challenges associated with data gravity, which refers to the increasing difficulty of moving data as it accumulates in size. Data fabric abstracts the intricate technical aspects involved in data transfer, conversion, and integration, ensuring the availability of all data throughout the entire enterprise. Data fabric architectures revolve around loosely connecting data within platforms to meet the requirements of applications. In a multi-cloud setting, for instance, a data fabric architecture might involve one cloud platform like AWS handling data ingestion, another platform like Azure managing data transformation and utilization, and a third vendor such as IBM Cloud Pak® for Data offering analytical services. The data fabric architecture interconnects these environments, creating a unified data perspective. Nationally and Internationally reputed school, Syracuse University began to experience financial stress due to the vast amount of data being inputted and stored in their systems. It became hard to manage. To combat this, they built a multi-cloud-data fabric. To manage the data fabric, the software team utilized NETAPP’S ONTAP, which enabled dynamic tiering: automated data routing to suitable storage tiers, determined by identified performance requirements and cost considerations. This intelligent functionality optimizes performance for crucial data and applications, minimizes storage expenses for less frequently accessed data, and lessens the workload for IT staff. The difference between Data Fabric and Data Mesh is that data fabric serves as a centralized system for the management of numerous data sources. In contrast, data mesh establishes multiple specialized systems designed for distinct functions and purposes.
Comments